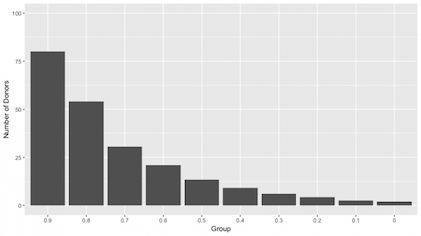
Predicting churn in a regular giving program
Chris Paver –
Dataro recently teamed up with a large humanitarian organisation to see how well we could predict attrition in a regular giving program. The results showed that machine learning methods can be used to predict attrition with a very high degree of accuracy, allowing charities to take proactive steps to engage with these important donors.
Why try to predict regular giver attrition?
Regular giving programs are the engine rooms driving many important causes. But when it comes to combating regular giver attrition, many charities are still using reactive strategies like decline calling programs. The problem with this approach is that the donor has already missed a payment or cancelled a gift before the charity is able to act. If charities can predict in advance which donors are the most likely to churn, they can take action to engage with those donors proactively to keep the relationship strong.
Setting up the test
The first challenge was to determine how effectively Dataro could predict attrition. So we decided to conduct a historical analysis using the following methodology:
- Our charity partner provided Dataro with historical data from its CRM up to 30 September 2018. The data included transactions, communications, demographic information and more. Critically, the charity withheld all data after that date, so Dataro could not see it.
- Dataro trained a series of ‘churn event’ models on the historic data to generate propensity scores on a scale of 0-1 for every regular giver. Donors with scores closer to 1 were considered more likely to churn. A ‘churn event’ included active cancellations and passive churn (3 missed payments in a row).
- Once the predictions were provided, our partner uploaded up to date data for the 6 months after 30 September 2019. We then compared our predictions against actual churn events during that period.
Analysing the results
The results showed the machine learning was highly effective for predicting regular giver churn. Critically:
- more than 75% of donors with scores of 0.9 – 1 had actually churned in the six month test window;
- there was a very strong correlation between prediction and actual churn events across all predictive bands;
- nearly 800 of the top 1000 scores actually churned in the six month test window.
These results mean the organisation could have a very high level of confidence that the donors with the highest scores were, in fact, the most likely to churn. A campaign directed towards these donors would therefore reach a high number of churners.
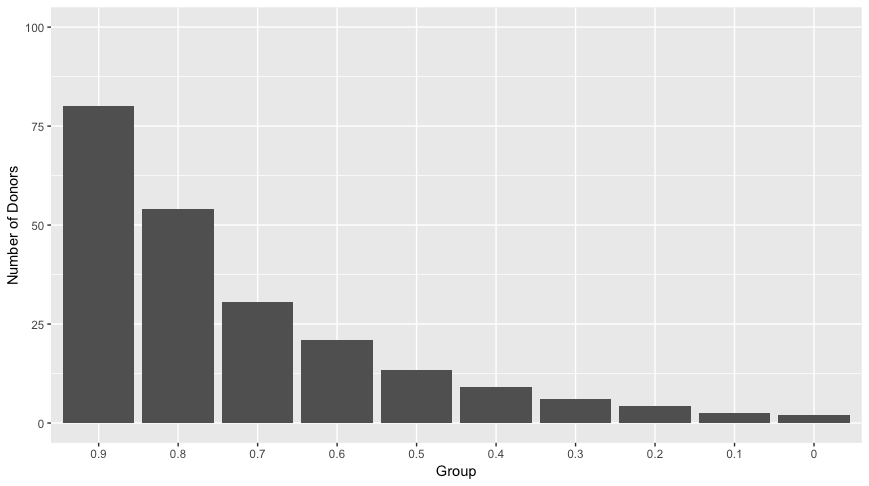
Next steps
Without predictive modelling, a charity has no way of knowing which donors are the most likely to churn beyond some basic assumptions. For example, an organisation may be aware that some donors, such as those acquired through face-to-face programs, tend to churn at a higher rate than others. But it has no way of knowing which of the donors will leave. And when it comes to loyal donors with more than 3 months tenure, understanding which are likely to leave becomes more challenging again.
The advantage of a predictive modelling approach is that charities have the option of testing different methods to improve retention by engaging with ‘at risk’ donors. This means they have the opportunity to address churn before it happens, rather than relying only on old reactive strategies.