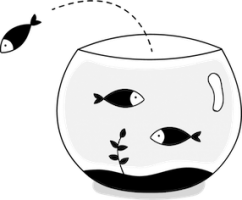
CASE STUDY: ActionAid Australia tested Dataro’s machine learning methods to help raise funds for women’s rights and social justice
Chris Paver – 11 November 2019
Dataro partnered with ActionAid Australia in mid-2019 to test whether machine learning could be used to support ActionAid’s critical mid-year tax appeal. The appeal is an important annual program, raising funds to empower women globally to claim their human rights and campaign against injustice.
The goal was to identify supporters with a higher ‘propensity to give’ in the tax appeal, allowing ActionAid to reduce mail volumes and save on campaign overheads. At the same time, using machine learning to identify patterns of behaviour could help ActionAid to engage with additional supporters who would otherwise fall outside their usual campaign selection criteria.
Dataro used machine learning algorithms to generate propensity scores from an analysis of transactions, communications, and demographics data. Each donor was given a score between 0 and 1 to reflect their propensity to give, with scores closer to 1 representing a higher probability of giving. Using these scores, ActionAid selected a substantially smaller campaign list than in previous years. The results showed a strong correlation between predictive scores and instances of giving, highlighting the utility of this new methodology.
Key results included:
- 73% of campaign revenue was donated by supporters with propensity scores above 0.8 (i.e. donors the analysis indicated had the highest propensity to give) and 15.2% of revenue was donated by supporters with propensity scores between 0.6 and 0.8. The scores were highly predictive of actual giving.
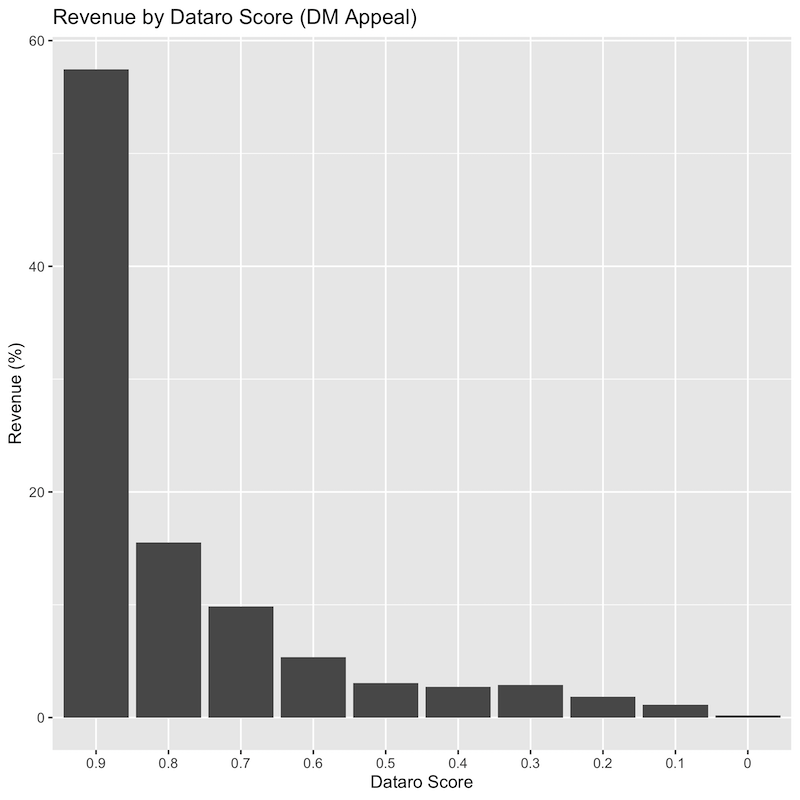
- Supporters with the highest propensity scores also had by far the highest response rates. For example, nearly 50% of donors with scores above 0.9 gave to the campaign, while 25% of donors with scores between 0.8 and 0.9 gave to the campaign. That compares with a response rate of about 1% for donors with scores of 0.2 or lower.
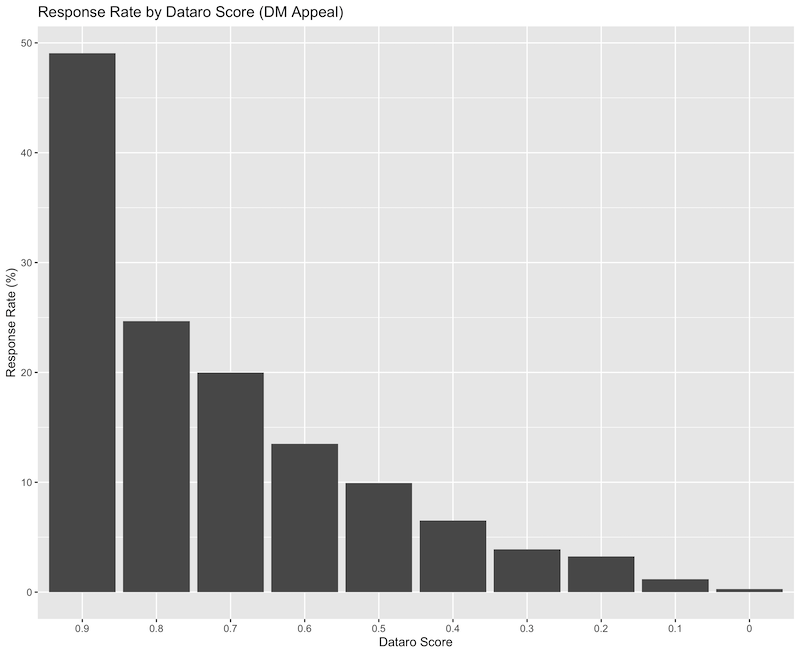
- Another interesting outcome was that the vast majority of financial gifts received during the campaign period that were not attributed directly to the appeal also came from donors with the highest propensity scores. This again validates the usefulness of the modelling process for predicting potential givers.
On the basis of these early results, ActionAid Australia is now working with Dataro to integrate machine learning propensity scoring directly into the organisation’s CRM. If you are interested in learning more about Dataro integrations, you can contact us here.