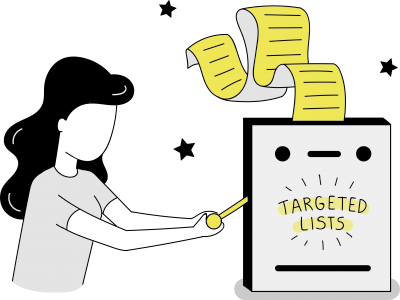
How to cut costs and increase returns on your tax appeal
Chris Paver – 1 March 2019
As the end of the financial year approaches, Australian households will be bombarded with requests for donations as charities and other organisations roll out their tax appeals.
The ability to claim a tax deduction is a strong incentive for some donors to give. It was estimated in 2016 that 28% of all gifts made by individuals were claimed as tax-deductible gifts, according to the Giving Australia report. But while the direct mail tax appeal is a critical campaign for many charities, it can also result in so much wasted paper and expense as charities struggle to hit their targets in a crowded market.
Heading into this year’s tax appeal is the perfect time for organisations to ask what what steps they are taking to improve their return on investment. Unfortunately, while classic tactics like personalised messages are all widely used, not-for-profits still lag behind their commercial counterparts when it comes to targeting and data.
Why use data for better targeting?
The goal of a targeted DM campaign is simple: to reduce wastage and improve ROI. The challenge is to know which donors to include and which to exclude in order to maximise returns. Traditional selection processes such as RFM (recency, frequency, monetary value) analysis can get organisations part of they way there, but still result in substantial campaign wastage and missed income. This is because they are based on the application of simplistic rules and broad assumptions (such as excluding all ‘lapsed’ donors), rather than individual, evidence-based scoring.
At Dataro, we think the solution lies in propensity modelling. Here’s how a data scientist might approach the problem. Rather than applying rudimentary selection rules, we use machine learning techniques to train a propensity model on the charity’s entire donor base. This process involves taking hundreds of factors (such as age, location, donation history, time of year, house or apartment, marriage status, etc), and then looking at the entire history of the organisation’s fundraising to discover how important each factor is and to provide ‘propensity scores’ for every individual donor on the database. That allows us to tell who is the most and least likely to give. You can read more about it here.
The first benefit of this approach is that the organisation can immediately exclude donors with a very low likelihood of responding to the campaign, reducing campaign costs. If the projected response rate is below the break-even point for the group, they can be excluded.
The second advantage is that better targeting results in improved response rates and therefore a higher campaign ROI. By using propensity modelling to identify those most likely to give, additional campaign tactics such as second wave DM or supplementary telemarketing contacts can be directed to the donors where they are likely to have the greatest impact, again increasing campaign effectiveness and minimising wastage.
How does it work?
Let’s look at the theory in a conservative campaign scenario. Here are our assumptions (using example figures only):
Campaign size: 50,000 DM targets
Average cost per DM: $1
Average gift: $50.
Using propensity modelling, every member of the database is given a personal propensity score based on hundreds of factors. In Dataro’s approach, people with a ‘Low’ or ‘Very Low’ score could be excluded from the campaign. The groups might look something like this: Very Low: 3000, Low: 8000, Moderate: 20,000, High: 13,000, Very High: 6000.
By excluding the ‘Very Low’ and ‘Low’ groups, savings of $11,000 are immediately made. The campaign is run targeting the remaining 39,000 potential donors.
The next step would be to calculate the required break-even response rate. Because we know our average gift in this example is $50, the required response rate to break even is 2% (B/E = Cost per piece / Average Gift). In other words, in order to break even on the campaign the organisation needs 780 people of the 39,000 mailed to respond (assuming an average gift of $50). The goal is to get a response rate of more than 2% in order to to maximise ROI, which is exactly what proper targeting using propensity modelling is designed to achieve.
By focussing on the people most likely to respond based on the organisation’s entire fundraising history (rather than simplistic rules), average response rates can increase significantly. Across campaign types, we would typically see the lowest response rate for those donors with a ‘very low’ score and the highest response rates for the ‘very high’ group.
Looking at our example again, conservative campaign response rates for a well-targeted campaign might look something like this:
Group | Response Rate |
Very High | 12% |
High | 8% |
Moderate | 4% |
Here’s how the campaign net revenue would look per group, again assuming a $50 average gift (Net revenue = Size of Group * Response Rate * Average Gift).
Group | Size | Response Rate | Average Gift | Net Revenue |
Very High | 6000 | 0.12 | 50 | 36,000 |
High | 13000 | 0.08 | 50 | 52,000 |
Moderate | 20000 | 0.04 | 50 | 40,000 |
TOTAL | 128,000 |
Calculating ROI
Now let’s calculate our ROI. Generally, we would expect a better ROI for higher scores.
ROI = (Net revenue) / (cost of campaign)
Group | Revenue | Cost | ROI |
Very High | 36,000 | 6000 | 6.0 |
High | 52,000 | 13,000 | 4.0 |
Moderate | 40,000 | 20,000 | 2.0 |
TOTAL | 128,000 | 39,000 | 3.28 |
This example demonstrates how the higher the propensity, the better the projected ROI from the campaign. On the other hand, lower propensity scores achieve a substantially reduced ROI (and in some cases a negative ROI). In an increasingly competitive and cluttered campaign period such as tax time appeals, taking a simple step like using propensity modelling rather than traditional selection processes has the potential to change the game for many organisations by limiting wastage and increasing campaign returns.